Unless you’ve been living off the grid the past year, it's unquestionable that artificial intelligence (AI) is the next big thing in tech. As of early 2023, ChatGPT is the tool everyone's talking about. A quick scroll through Twitter will show you people raving about its capabilities, from writing scripts, fixing code, generating recipes to even engaging ChatGPT in deep and thought-provoking discussions on existential questions. It is clear that AI has woven itself into the fabric of our daily lives, reshaping industries and redefining possibilities.
But ChatGPT is just the tip of the iceberg in a vast ocean of AI-driven innovation. As we enter this era of AI, the integration of artificial intelligence (AI) into product development has become increasingly prevalent. But how do you build AI (or AI-powered) products that people want and are of utility?
Despite its potential, building AI-powered products requires a nuanced approach that differs from traditional product development strategies. In a recent webinar hosted by Wizly, we invited AI product strategist Dr. Eva Agapaki (Founder of Hatch Labs)to share her insights on how to create impactful, AI-driven products. Eva has a decade’s experience in evaluating AI ideas, launching new AI products to market and scaling high growth startups. In her tenure as an AI Professor at the University of Florida, she validated early-stage ideas and partnered with cross-functional teams, which resulted in generating a $1.5M research portfolio.
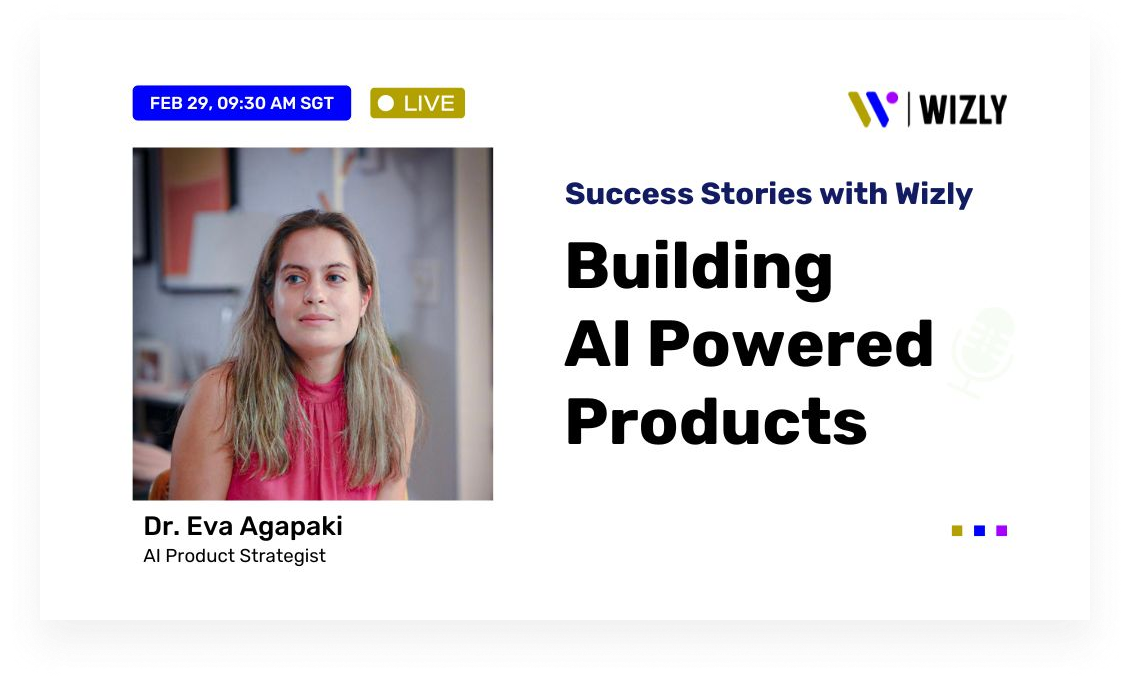
Below, we summarise key takeaways and practical examples from her discussion.
Developing AI-driven products: Lessons from failures
There are many myths and misconceptions surrounding the use of AI in product development. Perhaps the biggest of all is the misconception that AI products follow the same principles as traditional products.
- The reality is, AI products require an entirely different launch strategy. AI products often rely on research-based approaches, necessitating a different launch strategy. Case studies like the failure of IBM Watson's “supercomputer” oncology project underscored the importance of setting clear product requirements from the outset. Initially hailed as a game-changer in medicine, Watson aimed to revolutionise cancer care by offering tailored treatment recommendations based on vast amounts of data. However, investigations reveal a sobering reality: Watson has struggled to meet the lofty expectations set by IBM, with significant limitations and challenges undermining its effectiveness.
- Novelty isn't a guarantee of product success. Dr. Agapaki challenged the idea that the novelty or "wow" factor of AI technology ensures success. Instead, she believes the emphasis should be on addressing real problems and providing tangible value to users. The downfall of Anki, a robotics and AI startup, serves as a warning. Despite initial success with its innovative toys, its sudden closure in 2019 shows that regardless of a product's novelty, it won't succeed without proving its value in the consumer market. This underscores the importance of functionality over novelty.
- Complexity is not superior. Dr. Agapaki often encounters the misconception that in AI technology, complexity equates to superiority. However, the truth is that user-friendliness and problem-solving effectiveness are more important. For instance, the Seven Dreamers Laboratory built a robot that could wash, iron, and fold laundry. While the idea was complex, the project failed because the product was expensive and couldn't match the dexterity of a human. This underscores the importance of setting realistic expectations and prioritising usability.
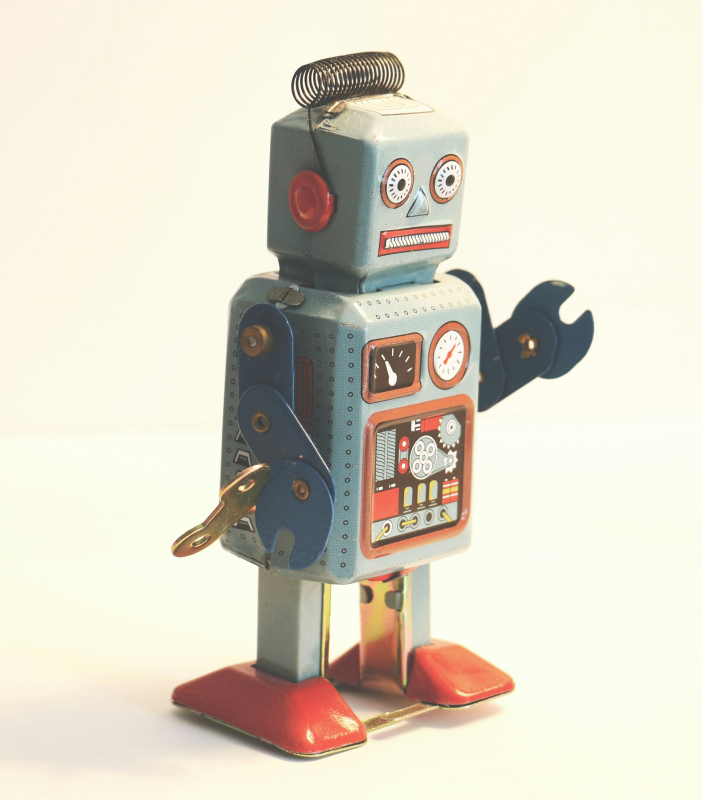
The Generative AI Flywheel
How can you develop an AI product that aligns with user needs? In her session, Dr. Eva introduces her Generative AI Flywheel. This framework consists of data, AI models, and user feedback. It's a strategy for using generative AI to drive innovation and solve problems across various fields.
Each part is vital in the product development process, promoting constant progress and a focus on the user. Dr. Eva's method includes a continuous cycle of generating, evaluating, and improving AI outputs to refine the results.

Here's an explanation of how the Generative AI Flywheel operates:
- Generation: The process starts with generating a diverse set of outputs using generative AI models. These models are trained on vast amounts of data and can produce outputs such as images, text, music, or other creative works. The generated outputs are often novel and can serve as inspiration for further exploration.
- Evaluation: Once the outputs are generated, they are evaluated by humans or other AI systems to assess their quality, relevance, and usefulness. Evaluation criteria may vary depending on the specific application, but generally involve assessing factors like creativity, coherence, and alignment with desired objectives.
- Iterative Improvement: Based on the evaluation feedback, adjustments and improvements are made to the generative AI models. This could involve fine-tuning the model's parameters, updating the training data, or modifying the generation process itself. The goal is to enhance the model's ability to produce high-quality outputs that better meet the desired criteria.
- Feedback Loop: The refined generative AI models are then used to generate a new set of outputs, restarting the cycle. With each iteration of the flywheel, the quality and relevance of the generated outputs improve, driving further innovation and exploration.
Case Studies: Successful Real-world Applications
Earlier, we shared examples of AI-driven innovation that failed to meet the expectations of customers. Conversely, what does a successful application of an AI product outlook like? During the session, Dr. Eva provided two compelling case studies of successful AI product implementation:
- Scale AI: This product exemplified the integration of data, AI models, and user feedback. By outsourcing data labelling tasks and incorporating rigorous validation processes, Scale AI streamlined product development and established partnerships with industry leaders.
- Mandrake: An MIT spinoff, Mandrake addressed the challenge of deploying ML models in real-time without GPUs. By simplifying model deployment processes and providing CPU performance through software, Mandrake enabled rapid deployment and scalability for enterprises like Smart Shopper.
How to get started with your AI product development
Dr. Eva concluded the webinar with practical strategies for achieving ROI in AI product development:
- Start Small: Begin with pilot projects or hackathons to validate ideas and garner feedback.
- Data-Driven Roadmaps: Prioritise features based on quantitative metrics rather than intuition.
- Simplicity and Scalability: Emphasise simplicity in product design to facilitate scalability and adoption.
- Crossing the Chasm: Differentiate between early adopters and mainstream customers to achieve widespread market acceptance.
Conclusion
In a rapidly evolving landscape, the fusion of artificial intelligence with product development opens new frontiers of innovation and opportunity. As businesses continue to harness the power of AI, a strategic and user-centric approach will be paramount in realising the full potential of AI-powered products.
Watch the full webinar replay here. Want to connect with Dr. Eva Agapaki? She is now available for projects and 1:1 calls on Wizly.